AI in 2025: What You Need to Know (No Hype, Just Facts!)
Are you excited or scared about AI?
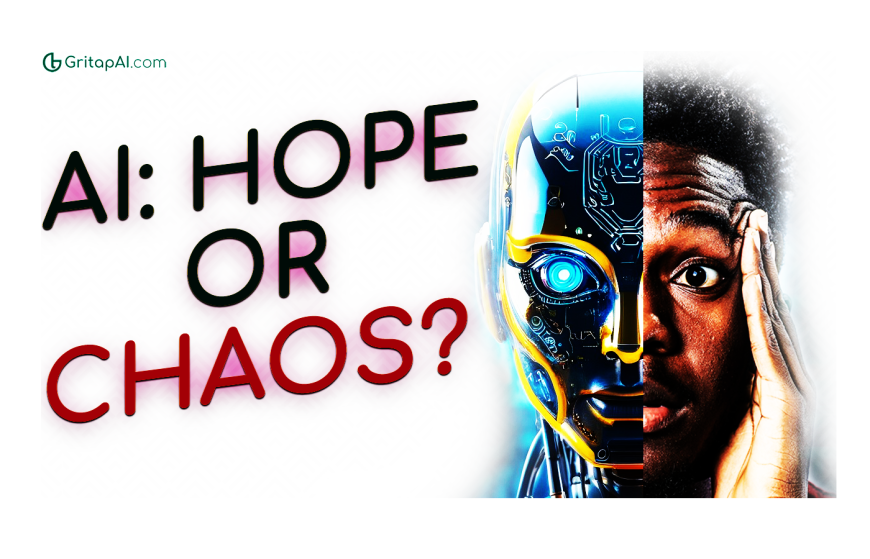
-
Introduction
AI (Artificial Intelligence) is basically the use of computers to do jobs/tasks that frequently require human intelligence through automated decision-making.
This particular field is dedicated to the idea of reproducing human abilities, including creativity, self-improvement, language use and many others.
The early forms of the AI operations we constantly use were built on traditional machine learning models. These models depend on algorithms drafted and managed by data scientists. In substance, traditional machine learning models need to reuse new data and address tasks that go beyond their original training.
At the moment, AI encompasses many subfields. Our understanding of both practical and theoretical AI is evolving, which means that AI terminologies or language can vary and occasionally overlap between different sources.
Nonetheless, the different types of AI can be distributed into two main areas: AI capabilities and AI functionalities.
.
-
What are the three kinds of AI grounded on capabilities?
-
Artificial Narrow Intelligence( ANI):
ANI, also referred to as Weak AI, is the only kind of AI that is currently in use. Any other form of AI is theoretical. It can be trained to perform a single or narrow task, often far more quickly than the human mind is capable of. Still, it can’t perform outside of its defined task, rather, it targets a single subset of capabilities of the mind and developments in that field. Apple's AI, Meta AI and Claude are examples of Narrow AI. Since ChatGPT by OpenAI is restricted to the specific task of the conversation, it is in fact also regarded as a type of narrow artificial intelligence.
.
-
Artificial General Intelligence( AGI):
Artificial General Intelligence( AGI), also known as Strong AI, is at the moment nothing further than a theoretical conception. Without the requirement for human training of the underlying models, AGI will leverage prior literacies and skills to navigate new tasks in a different environment. Because of this skill, AGI will be able to learn and carry out any intellectual work that a person is capable of.
.
-
Artificial Super Intelligence( ASI):
The term "Super AI" usually refers to Artificial Super Intelligence and, like AGI, is theoretical. If Super AI were to be developed, it would, presumably, reason. The operations that benefit from super AI skills will have advanced past the point where they can comprehend human emotions and gestures, feel emotions, have needs, and hold onto their own beliefs and requests.
.
-
-
What are the four types of AI grounded on functionalities?
-
Reactive Machine AI
These are AI systems with no memory and are designed to perform an extremely specific task. Since they can’t recollect former issues or opinions, they only work with presently available data. Reactive AI is based on statistical computation and can analyze large amounts of data to create a seemingly thoughtful conclusion.
Example of Reactive Machine AI
- The recommendation engine on Netflix Netflix uses models to analyze large datasets of past viewing behavior to suggest material to users based on what they're most likely to appreciate..
-
Limited Memory AI
Unlike Reactive Machine AI, This type of AI has the ability to remember past
occurrences, problems, and analyze particular items or circumstances over time.
In order to decide on the optimal course of action for reaching a particular goal, Limited Memory AI can analyse both previous and current data.
Even yet, Limited Memory AI is only able to use one piece of data at a time; it is unable to store multiple pieces of data in a library for extended periods of time. Limited Memory AI's performance can improve over time as it has more experience and is taught on more data.
-
Examples of Limited Memory AI
-
i ) - Generative AI:
ChatGPT, Meta AI, and Gemini are examples of generative AI systems that use limited memory AI capabilities to predict the next word, expression, or visual aspect in the content they are creating.
-
ii ) - Virtual assistants and chatbots:
Chatbots such as Siri, Alexa and Google Assistant combine natural language processing( NLP) and Limited Memory AI to understand questions and requests, take applicable conduct and compose responses.
.
-
-
Theory of Mind AI
This is a functional class of AI that falls underneath the General AI. Though an unrealized form of AI at the moment, it would be able to comprehend the emotions and research of different worlds. This would, in theory, enable the AI to mimic human-like connections. It would represent its relationships with individualities based on their distinct emotional needs and goals since it could deduce mortal motives and logic.
Theory of Mind AI would also be suitable to interpret and contextualize essays and artwork, something that current generative AI technologies are unfit for.
Researchers studying artificial intelligence (AI) think the technology will be able to analyze sounds, images, and other types of data to mimic, pretend, hide, and react emotionally to humans.
To date, Emotion AI is unfit to comprehend and react to human passions.
.
-
Self-Aware AI
This is a kind of functional AI class for operations that would retain super AI capabilities. Like the Theory of mind AI, Self-Aware AI is strictly theoretical. However, it would have the capability to understand its own internal conditions and traits along with human feelings and studies, If ever achieved. It would also have its own set of feelings, requirements and beliefs.
.
-
-
What are the 4 pillars of Machine Learning?
AI, or artificial intelligence, broadly speaking, refers to machines that think and behave like humans. Machine learning (ML), a branch of artificial intelligence, has developed methods that could allow computers to learn without clear guidance/instructions.
In order to create intelligent machines and hence achieve artificial intelligence, machine learning (ML) practitioners—such as AI scientists, engineers, technicians, and others—must integrate a number of ML techniques.
In fact, when considered separately, every learning technique that relies on gaining knowledge from data examples—like the Supervised Learning technique—is a machine learning approach.
.
We give a thorough rundown of each ML pillar in this section, which is now the most popular form of AI.
-
Supervised Learning (SL)
The Supervised Learning (SL) technique in machine learning involves learning via labeled samples.
Essentially, the process involves instructing a model—behaving like a student—on how to choose the right response or output for a given input. As a result, each data set that the teacher provides is divided into two sections: features for the input and labels for the output. The values we want the model to predict are called labels.
Pattern Recognition is comparable to Supervised Learning.
We learn a Conditional Probability Distribution P(Y|X) when the mapping from input (x) to output (y) via a pattern function f is stochastic (not close to an affine function of elements of the training set). The challenge of learning is referred to as Classification when the output y falls inside a limited range of values. Regression is the learning problem when the outcome, y, is a number.
.
-
Unsupervised Learning (UL)
The Unsupervised Learning (UL) technique uses clusters—categorized groups of related objects or cluster analysis—to learn from unlabelled samples. Without a label, the UL model classifies the given data automatically. It does, however, exist in different forms. First, there is the Self-Supervised Learning subset of UL, which depends on object encoding rather than clustering and grouping as its primary focus. The next type of learning is called semi-supervised learning, and it involves using both labelled and unlabelled data;It combines elements of both SL and UL techniques.
.
-
Reinforcement Learning (RL)
The term Reinforcement Learning (RL) refers to a Behaviour Learning approach that is based on a World model (model-based) or a Mathematical model (model-free). It essentially relies on a kind of reward system in which the RL model (the agent) always seeks to maximize or optimize reward value in accordance with a given strategy (the policy) that instructs the agent on how to behave. Nevertheless, there are situations when a suboptimal value rather than the maximum value of the stated reward achieves the system's ideal performance. We assume no prior knowledge of the reward function or the environment in reinforcement learning. For a Passive Reinforcement Learning scheme, we only consider a fixed policy of state-action pairs. For an Active Reinforcement Learning scheme, we also consider a self-decision-making approach regarding what to do.
.
-
Deep Learning (DL)
The process of learning using inductive Connectionist or Brainlike Layered models is known as deep learning (DL). Here, the learning process is repeatedly running the data through the DL model, providing feedback and adjusting the parameters (backpropagation) until the model reaches a steady state that may be suitable for inference (i.e., for use in real-world applications).
Since deep learning (DL) technology is a structural replica of the way the human brain functions (in general) and is widely applied to machine learning techniques, we shall go further into it.
A number of AI concepts that AI scientists adopted were focused on developing a brain language by examining its physical characteristics in order to tackle AI.
Please take note that language refers to the internal processes of the brain that allow it to express itself or respond to the outside world.
For them, building a model that replicates every aspect of the biological brain is building an artificial brain model. Considering their diverse methods for developing a brain language, we can conclude that researchers in artificial intelligence (AI) develop machine intelligence by observing brain functions—behavioral, intentional, or brain states—all of which are produced by the same biological brain. In fact, developing a sufficiently trustworthy language from the biological brain may result from a careful examination of the facts obtained from the observation of brain functions.
Does this imply, therefore, that the artificial brain languages (models developed from the study of the human brain) are the true biological brain language, or the precise means by which the human brain actually communicates?
We're not entirely certain.
In contrast, the artificial neuron's connections are binary rather than analogical. There is only one binary link between two (02) artificial neurones. However, because of our current computer capability, each artificial neurone might be coupled to a relatively small number of other neurons—less than 10,000.
Through a variety of topologies, Artificial Neural Networks (ANN) can simulate the functioning of the human brain and aid in the development of artificial intelligence.
However, even if these apps simulate human brain functions (behavioral, purposeful, or brain states), they are not the exact means by which the human brain communicates and so are not the real human brain language.
The artificial brain's language cannot, in fact, be a perfect replica of the biological brain's own language because of the differences in the biological brain's connections and internal linkages.
Naturally, artificial brain language functions effectively and replicates key aspects of human intelligence. It may even surpass humans in some tasks, but we are not the same species (we believe that certain specificities will only be achievable by humans). The biological human brain imposes dimensions of data that are unknown and unexamined, which the artificial brain overlooks.
Understanding these branches helps us grasp where technologies like Large Language Models fit in and how they contribute to the broader field of AI..
-
-
AI's Current Capabilities
So, what can AI do at the moment?
1. Natural Language Processing ( NLP ):
This is AI's capacity to comprehend and produce human language. Applications include automated chatbots for customer support, virtual assistants like Siri and Alexa, and even programs that aid with writing code or emails.
2. Computer Vision:
This area of artificial intelligence enables machines to comprehend and decide on the basis of visual data. It is utilised in medical imaging, driverless cars, facial identification, and even smartphone cameras to improve picture quality.
3. Predictive Analytics:
Artificial Intelligence is very good at analysing actual facts to forecast future events. This is applied in a number of industries, including marketing, healthcare, finance, and healthcare (e.g., disease outbreak prediction, and consumer behaviour prediction).
.
-
AI's Current Limitations
It's crucial to acknowledge that AI has its limitations. NLP is impressive, but it still has trouble understanding linguistic context.
Despite its strength, computer vision is susceptible to bias and readily tricked. Due to the fact that machine learning (ML) algorithms rely on pre-existing data, marginalised groups who have experienced systemic and human biases may receive inaccurate projections or have certain resources withheld from them.
As they say, "garbage in, garbage out" applies to predictive analytics, which is only as good as the data it is trained on.
Even while AI and ML have many advantages, there are worries about the hazards and difficulties that black-box ML models may present.
When decision-makers are unaware of the reasons behind the current results and are unsure of how the system will generate results in the future, they find it difficult to trust the procedures.
.
-
Conclusion
By understanding the description, elaboration, and current capabilities of AI, we can better estimate whether AI is truly as revolutionary as it's claimed to be.
Comment below some interesting everyday examples around you where machines are learning and doing amazing jobs in your country.